text파일 다루기
%%writefile test.txt
Hello, this ih a quick test file.
This is the Second line of the file.
Writing test.txt
pwd
'/content'
my_file = open('test.txt')
my_file = open('/content/test.txt')
my_file
<_io.TextIOWrapper name='/content/test.txt' mode='r' encoding='UTF-8'>
my_file.read()
'\nHello, this ih a quick test file.\nThis is the Second line of the file.'
my_file.read() # 파일을 한번 읽어들이면 다음에는 안나와요
''
my_file.seek(0) # 다시 앞으로 와서 읽을 수 있어요
0
my_file.read()
'\nHello, this ih a quick test file.\nThis is the Second line of the file.'
# readlines() : 개행 문자를 기준으로 모든 줄을 읽어서 한 라인씩 리스트로 값을 반환
my_file.seek(0)
my_file.readlines()
my_file.close() # 열었으면 닫아주세요
my_file = open('test.txt', 'w+')
my_file
<_io.TextIOWrapper name='test.txt' mode='w+' encoding='UTF-8'>
my_file.read() # w+를 사용하면 덮어쓰기로 실행되서 아무것도 안나와요.
''
my_file.write('This is a new first line')
24
my_file.seek(0) # 포지션 다시 0번
my_file.read()
'This is a new first line'
my_file.close()
my_file = open('test,txt','a+')
my_file.write('\nThis line is being appended to test.txt')
my_file.write('\nAnd another line here')
22
# r 읽기모드 - 파일을 읽기만 할 때 사용
# w 쓰기모드 - 파일에 내용을 쓸 때 사용
# a 추가모드 - 파일의 마지막에 새로운 내용을 추가 시킬 때 사용
# 업데이트 모드라고도 하는 +기존 열기 모드에 읽기 또는 쓰기를 추가합니다.
# r수단 reading파일 ; r+파일을 의미 reading and writing합니다.
# w수단 writing파일 ; w+파일을 의미 reading and writing합니다.
# a수단 파일 , writing추가 모드; 파일, 추가 모드를 a+의미 합니다.reading and writing
my_file.seek(0)
print(my_file.read())
This is a new first line is a new first lineneThis is a new first line
with open('test.txt', 'r') as txt:
first_line = txt.readlines()[0]
print(first_line)
This is a new first line is a new first lineneThis is a new first line
# 파일에 저장된 모든 라인을 출력하시오.
with open('test.txt', 'r') as txt:
for line in txt:
print(line, end='')
This is a new first line
PDF파일 다루기
대충 이렇게 생긴 2페이지 위키백과 PDF 파일
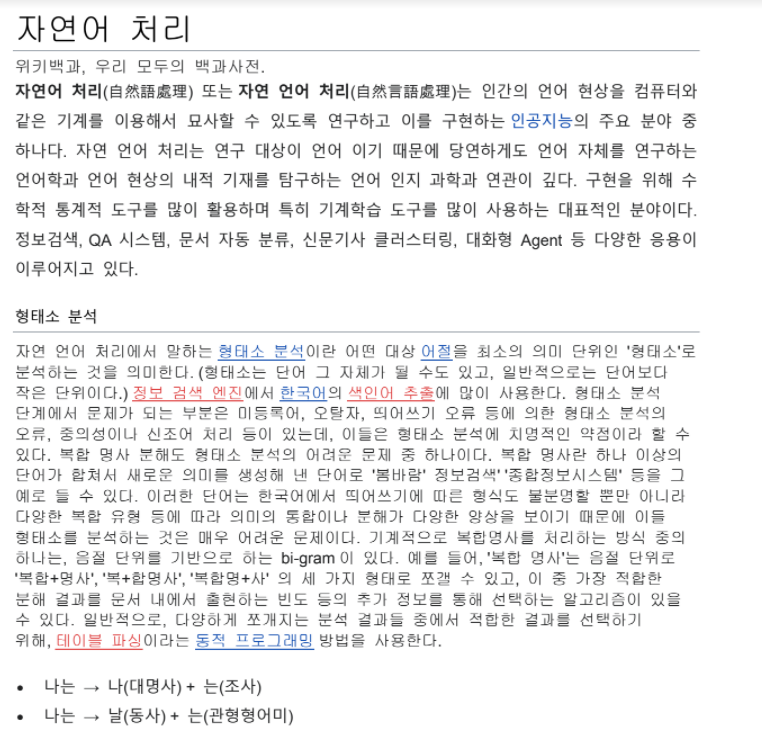
pip install pypdf2 # PDF파일 다루는 Module
import PyPDF2
!pip install tika # 한글 깨짐 방지
f = open('/content/drive/MyDrive/2203/KR_nlp.pdf', 'rb',)
pdf_reader = PyPDF2.PdfFileReader(f)
pdf_reader.numPages
2
page_one = pdf_reader.getPage(0)
page_one_text = page_one.extractText() # 텍스트만 추출
page_one_text
'8Ä7$6è\n\n=Ì*à\n \n88AØ-e˚0\n, \n7ä*à\n ...n, \n8¨&˘8t\n \n\n \"
from tika import parser # 한글 깨짐 방지
data = parser.from_file("/content/drive/MyDrive/2203/KR_nlp.pdf")
data
{'content': "\n\n\n\n\n\n\n\n\n\n\n\n\n\n\n\n\n\n\n\n\n\n\n\n\n\n\n\n\n\n\n\n\n\n\n\n\n\n\n\n\n\n\n\n\n\n\n자연어 처리 \n위키백과, 우리 모두의 백과사전. \n\n자연어 처리(自然語處理) 또는 자연 언어 처리(自然言語處理)는 인간의 언어 현상을 컴퓨터와 \n\n같은 기계를 이용해서 묘사할 수 있도록 연구하고 이를 구현하는 인공지능의 주요 분야 중 \n\n하나다. 자연 언어 처리는 연구 대상이 언어 이기 때문에 당연하게도 언어 자체를 연구하는 \n\n언어학과 언어 현상의 내적 기재를 탐구하는 언어 인지 과학과 연관이 깊다. 구현을 위해 수\n\n학적 통계적 도구를 많이 활용하며 특히 기계학습 도구를 많이 사용하는 대표적인 분야이다. \n\n정보검색, QA 시스템, 문서 자동 분류, 신문기사 클러스터링, 대화형 Agent 등 다양한 응용이 \n\n이루어지고 있다. \n\n형태소 분석 \n\n자연 언어 처리에서 말하는 형태소 분석이란 어떤 대상 어절을 최소의 의미 단위인 '형태소'로 \n\n분석하는 것을 의미한다. (형태소는 단어 그 자체가 될 수도 있고, 일반적으로는 단어보다 \n\n작은 단위이다.) 정보 검색 엔진에서 한국어의 색인어 추출에 많이 사용한다. 형태소 분석 \n\n단계에서 문제가 되는 부분은 미등록어, 오탈자, 띄어쓰기 오류 등에 의한 형태소 분석의 \n\n오류, 중의성이나 신조어 처리 등이 있는데, 이들은 형태소 분석에 치명적인 약점이라 할 수 \n\n있다. 복합 명사 분해도 형태소 분석의 어려운 문제 중 하나이다. 복합 명사란 하나 이상의 \n\n단어가 합쳐서 새로운 의미를 생성해 낸 단어로 '봄바람' 정보검색' '종합정보시스템' 등을 그 \n\n예로 들 수 있다. 이러한 단어는 한국어에서 띄어쓰기에 따른 형식도 불분명할 뿐만 아니라 \n\n다양한 복합 유형 등에 따라 의미의 통합이나 분해가 다양한 양상을 보이기 때문에 이들 \n\n형태소를 분석하는 것은 매우 어려운 문제이다. 기계적으로 복합명사를 처리하는 방식 중의 \n\n하나는, 음절 단위를 기반으로 하는 bi-gram이 있다. 예를 들어, '복합 명사'는 음절 단위로 \n\n'복합+명사', '복+합명사', '복합명+사' 의 세 가지 형태로 쪼갤 수 있고, 이 중 가장 적합한 \n\n분해 결과를 문서 내에서 출현하는 빈도 등의 추가 정보를 통해 선택하는 알고리즘이 있을 \n\n수 있다. 일반적으로, 다양하게 쪼개지는 분석 결과들 중에서 적합한 결과를 선택하기 \n\n위해, 테이블 파싱이라는 동적 프로그래밍 방법을 사용한다. \n\n\uf0b7 나는 → 나(대명사) + 는(조사) \n\n\uf0b7 나는 → 날(동사) + 는(관형형어미) \n\n품사 부착 \n\n형태소 분석을 통해 나온 결과 중 가장 적합한 형태의 품사를 부착하는 것을 말한다. \n\n보통 태거라고 하는 모듈이 이 기능을 수행한다. 이는 형태소 분석기가 출력한 다양한 분석 \n\n결과 중에서 문맥에 적합한 하나의 분석 결과를 선택하는 모듈이라 할 수 있다. 분석 시 \n\n문맥 좌우에 위치한 중의성 해소의 힌트가 되는 정보를 이용해서 적합한 분석 결과를 \n\n선택한다. 보통 태거는 대규모의 품사부착 말뭉치를 이용해서 구현하는데 은닉 마르코프 \n\n모델(HMM)이 널리 사용되고 있다. \n\n'나는'이라는 어절에 대한 형태소 분석이 다음과 같다면 \n\n\uf0b7 나는 → 나(대명사) + 는(조사) \n\n\uf0b7 나는 → 날(동사) + 는(관형형어미) \n\nhttps://ko.wikipedia.org/wiki/%EC%9D%B8%EA%B3%B5%EC%A7%80%EB%8A%A5\nhttps://ko.wikipedia.org/wiki/%ED%98%95%ED%83%9C%EC%86%8C_%EB%B6%84%EC%84%9D\nhttps://ko.wikipedia.org/wiki/%EC%96%B4%EC%A0%88\nhttps://ko.wikipedia.org/w/index.php?title=%EC%A0%95%EB%B3%B4_%EA%B2%80%EC%83%89_%EC%97%94%EC%A7%84&action=edit&redlink=1\nhttps://ko.wikipedia.org/wiki/%ED%95%9C%EA%B5%AD%EC%96%B4\nhttps://ko.wikipedia.org/w/index.php?title=%EC%83%89%EC%9D%B8%EC%96%B4_%EC%B6%94%EC%B6%9C&action=edit&redlink=1\nhttps://ko.wikipedia.org/w/index.php?title=%ED%85%8C%EC%9D%B4%EB%B8%94_%ED%8C%8C%EC%8B%B1&action=edit&redlink=1\nhttps://ko.wikipedia.org/wiki/%EB%8F%99%EC%A0%81_%EA%B3%84%ED%9A%8D%EB%B2%95\nhttps://ko.wikipedia.org/w/index.php?title=%ED%83%9C%EA%B1%B0&action=edit&redlink=1\nhttps://ko.wikipedia.org/wiki/%EB%AA%A8%EB%93%88\nhttps://ko.wikipedia.org/w/index.php?title=%EC%A4%91%EC%9D%98%EC%84%B1&action=edit&redlink=1\nhttps://ko.wikipedia.org/wiki/%EC%9D%80%EB%8B%89_%EB%A7%88%EB%A5%B4%EC%BD%94%ED%94%84_%EB%AA%A8%EB%8D%B8\nhttps://ko.wikipedia.org/wiki/%EC%9D%80%EB%8B%89_%EB%A7%88%EB%A5%B4%EC%BD%94%ED%94%84_%EB%AA%A8%EB%8D%B8\n\n\n다음과 같이 적절한 품사를 부착하는 것이 품사 부착이다. \n\n\uf0b7 나는 오늘 학교에 갔다' → '나(대명사)+는(조사) 오늘 학교+에 가다+았+다' \n\n\uf0b7 하늘을 나는 새를 보았다' → '하늘+을 날(동사)+는(관형형어미) 새+를 보다+았+다' \n\n구절 단위 분석 \n\n\uf0b7 구 단위 분석은 명사구, 동사구, 부사구 등의 덩어리를 의미한다. \n\no 서울시 서초구 서초동에 있는 가장 유명한 회사는 어디인가요? → 서울시 서초구 \n\n서초동에 있는 가장 유명한 회사는 어디인가요? \n\no 이 해결책은 정말이지 여기에는 적합하지 않아 → 이 \n\n해결책은 정말이지 여기에는 적합하지 않아 \n\n\uf0b7 절 단위 분석은 중문, 복문 등의 문장을 단문 단위로 분해하는 역할을 수행한다. \n\no 이 영화는 재미있었는데, 저 영화는 흥미 없었다 → 이 영화는 재미있었는데 , 저 \n\n영화는 흥미 없었다 \n\no 어제 내가 본 그 영화는 아주 재미있었다 → 어제 내가 본 그 영화는 아주 \n\n재미있었다. \n\no 나는 오늘 하늘을 나는 새를 보았다 → 나는 오늘 하늘을 나는 새를 보았다 \n\n이와 같이, 구 단위 분석을 먼저 수행하고 절 단위 분석을 해서 보다 큰 단위로 만든다. \n\n이러한 분석은 다음 단계인 구문 분석에서의 중의성을 해소하는 데 아주 중요한 역할을 \n\n수행한다고 할 수 있다. \n\n \n\nhttps://ko.wikipedia.org/wiki/%EA%B5%AC_(%EC%96%B8%EC%96%B4%ED%95%99)\nhttps://ko.wikipedia.org/wiki/%EC%A0%88_(%EC%96%B8%EC%96%B4%ED%95%99)\nhttps://ko.wikipedia.org/wiki/%EC%A4%91%EA%B5%AD%EC%96%B4\nhttps://ko.wikipedia.org/w/index.php?title=%EB%B3%B5%EB%AC%B8&action=edit&redlink=1\nhttps://ko.wikipedia.org/w/index.php?title=%EB%8B%A8%EB%AC%B8&action=edit&redlink=1\nhttps://ko.wikipedia.org/wiki/%EA%B5%AC%EB%AC%B8_%EB%B6%84%EC%84%9D\n\n",
'metadata': {'Author': 'user',
'Content-Type': 'application/pdf',
'Creation-Date': '2022-03-11T05:12:28Z',
..
..
'resourceName': "b'KR_nlp.pdf'",
'xmp:CreatorTool': 'Microsoft® Word 2016',
'xmpTPg:NPages': '2'},
'status': 200}
content = data['content'].strip()
content
'자연어 처리 \n위키백과, 우리 모두의 백과사전. \n\n자연어 처리(自然語處理) 또는 자연 언어 처리(自然言語處理)는 인간의 언어 현상을 컴퓨터와 \n\n같은 기계를 이용해서 묘사할 수 있도록 연구하고 이를 구현하는 인공지능의 주요 분야 중 \n\n하나다. 자연 언어 처리는 연구 대상이 언어 이기 때문에 당연하게도 언어 자체를 연구하는 \n\n언어학과 언어 현상의 내적 기재를 탐구하는 언어 인지 과학과 연관이 깊다. 구현을 위해 수\n\n학적 통계적 도구를 많이 활용하며 특히 기계학습 도구를 많이 사용하는 대표적인 분야이다. \n\n정보검색, QA 시스템, 문서 자동 분류, 신문기사 클러스터링, 대화형 Agent 등 다양한 응용이 \n\n이루어지고 있다. \n\n형태소 분석 \n\n자연 언어 처리에서 말하는 형태소 분석이란 어떤 대상 어절을 최소의 의미 단위인 '형태소'로 \n\n분석하는 것을 의미한다. (형태소는 단어 그 자체가 될 수도 있고, 일반적으로는 단어보다 \n\n작은 단위이다.) 정보 검색 엔진에서 한국어의 색인어 추출에 많이 사용한다. 형태소 분석 \n\n단계에서 문제가 되는 부분은 미등록어, 오탈자, 띄어쓰기 오류 등에 의한 형태소 분석의 \n\n오류, 중의성이나 신조어 처리 등이 있는데, 이들은 형태''''
with open("output.txt", 'w', encoding="utf-8") as txt:
print(content, file=txt)
Quiz
f-Strings
1.제공된 변수를 사용하여 NLP stands for Natural Language Processing 문자열 표시하시오.
abbr = 'NLP'
full_text = 'Natural Language Processing'
# Enter your code here:
print(f'{abbr} stands for {full_text}')
NLP stands for Natural Language Processing
Files
2. 다음 셀을 실행하여 현재 작업 디렉토리에 contacts.txt라는 파일 생성하시오.
%%writefile contacts.txt
First_Name Last_Name, Title, Extension, Email
Writing contacts.txt
3. 파일을 열고 read()를 사용하여 파일 내용을 'fields'라는 문자열에 저장하고, 파일이 마지막에 닫혀 있는지 확인하시오.
# Write your code here:
with open('contacts.txt') as c:
fields = c.read()
# Run fields to see the contents of contacts.txt:
fields
'First_Name Last_Name, Title, Extension, Email'
Working with PDF Files
4. PyPDF2를 사용하여 Business_Proposal.pdf 파일을 열고, 2페이지의 텍스트를 추출하시오.
# Perform import
import PyPDF2
# Open the file as a binary object
f = open('/content/drive/MyDrive/2203/Business_Proposal.pdf', 'rb')
# Use PyPDF2 to read the text of the file
pdf_reader = PyPDF2.PdfFileReader(f)
# Get the text from page 2 (CHALLENGE: Do this in one step!)
page_two_text = pdf_reader.getPage(1).extractText()
# Close the file
f.close()
# Print the contents of page_two_text
print(page_two_text)
AUTHORS:
Amy Baker, Finance Chair, x345, abaker@ourcompany.com
Chris Donaldson, Accounting Dir., x621, cdonaldson@ourcompany.com
Erin Freeman, Sr. VP, x879, efreeman@ourcompany.com
5. 추가 모드(Append)에서 contacts.txt 파일을 열고, contacts.txt에 위에서 2페이지의 텍스트를 추가하시오.
CHALLENGE: See if you can remove the word "AUTHORS:"
# Simple Solution:
with open('contacts.txt', 'a+') as c:
c.write(page_two_text)
c.seek(0)
print(c.read())
First_Name Last_Name, Title, Extension, EmailAUTHORS:
Amy Baker, Finance Chair, x345, abaker@ourcompany.com
Chris Donaldson, Accounting Dir., x621, cdonaldson@ourcompany.com
Erin Freeman, Sr. VP, x879, efreeman@ourcompany.com
page_two_text
'AUTHORS:\n \nAmy Baker, Finance Chair, x345, abaker@ourcompany.com\n \nChris Donaldson, Accounting Dir., x621, cdonaldson@ourcompany.com\n \nErin Freeman, Sr. VP, x879, efreeman@ourcompany.com\n \n'
# CHALLENGE Solution (re-run the %%writefile cell above to obtain an unmodified contacts.txt file):
with open('contacts.txt', 'a+') as c:
c.write(page_two_text[8:])
c.seek(0)
print(c.read())
First_Name Last_Name, Title, Extension, EmailAUTHORS:
Amy Baker, Finance Chair, x345, abaker@ourcompany.com
Chris Donaldson, Accounting Dir., x621, cdonaldson@ourcompany.com
Erin Freeman, Sr. VP, x879, efreeman@ourcompany.com
Amy Baker, Finance Chair, x345, abaker@ourcompany.com
Chris Donaldson, Accounting Dir., x621, cdonaldson@ourcompany.com
Erin Freeman, Sr. VP, x879, efreeman@ourcompany.com
Regular Expressions
6. 위에서 생성한 page_two_text 변수를 사용하여 Business_Proposal.pdf 파일에 포함된 이메일 주소를 추출하시오.
import re
# Enter your regex pattern here. This may take several tries!
pattern = r'[\w]+@[\w]+.[\w]{3}'
re.findall(pattern, page_two_text)
['abaker@ourcompany.com',
'cdonaldson@ourcompany.com',
'efreeman@ourcompany.com']
'자연어처리(NLP)' 카테고리의 다른 글
Word Embedding, Word2Vec (0) | 2022.03.22 |
---|---|
Tokenization (0) | 2022.03.22 |
베이즈 정리(Bayes’ theorem) (0) | 2022.03.21 |
정규표현식(Regular Expression) (0) | 2022.03.15 |
댓글